Realm for Cybersecurity
Cybersecurity Challenges in the AI Era
As the digital world expands, so do the cybersecurity threats. The rapid adoption of big data, AI, and large language models (LLMs) has introduced new risks related to data privacy, regulatory compliance, and AI-driven vulnerabilities. Organizations must navigate complex security challenges, including data integration hurdles, rising privacy regulations, and the potential exploitation of unstructured text data. Securing AI systems while maintaining transparency and compliance is now a mission-critical objective.
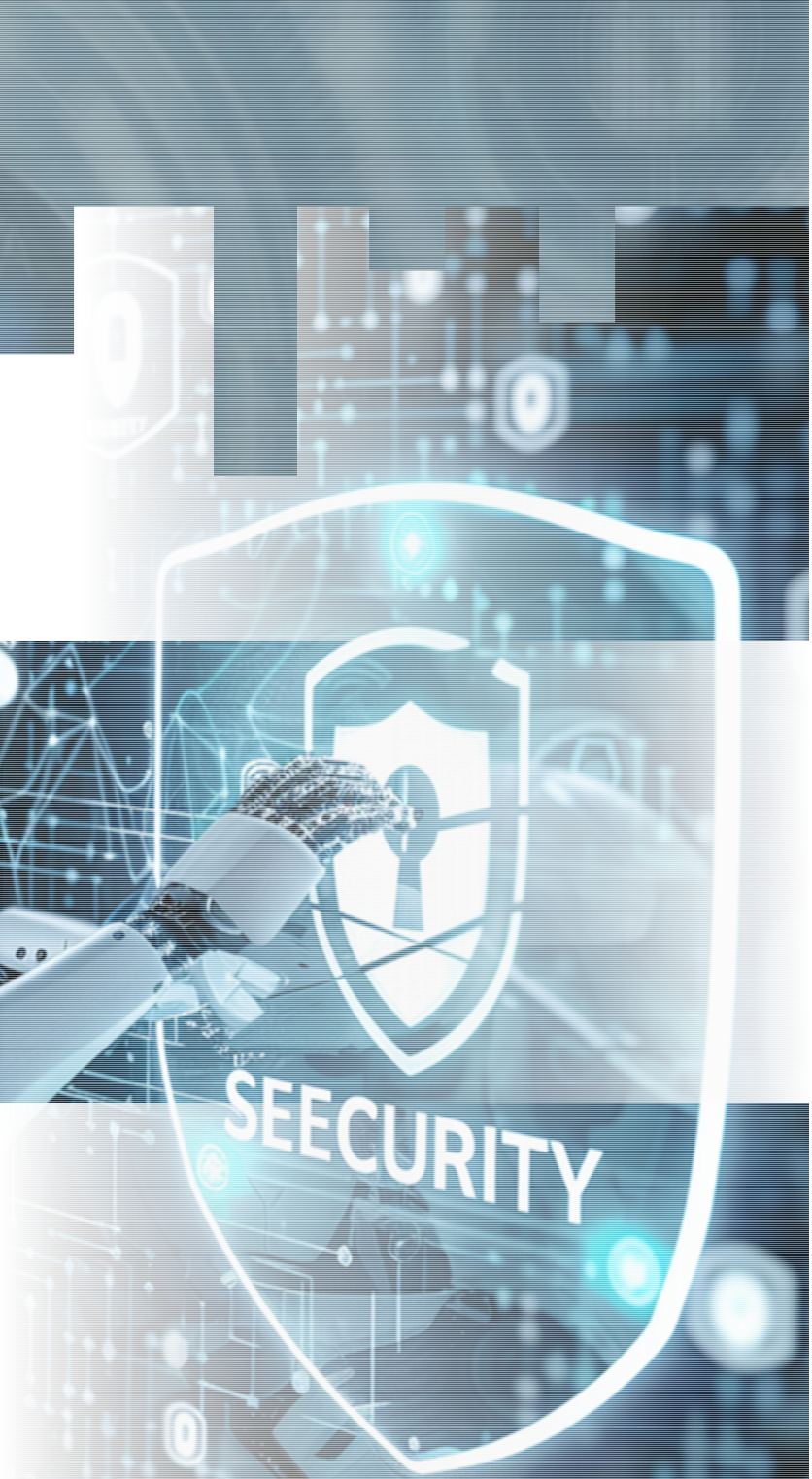
Rising Importance of Privacy in the Big Data Era
The massive growth of personal and corporate data makes privacy a key cybersecurity concern. PAMOLA’s synthetic data generation and differential privacy solutions enable organizations to process sensitive data without exposing real information, ensuring compliance with **GDPR, CCPA, and emerging AI regulations**.
Complexity of Data Integration and AI Security
Integrating distributed and siloed data sources without security risks is a major challenge. PAMOLA’s federated learning (FL) and secure multi-party computation (SMPC) allow AI models to be trained collaboratively across organizations **without raw data exchange**, preventing breaches while preserving analytical value.
Stricter Privacy Regulations & Compliance Risks
New AI regulations are reshaping data governance and compliance expectations. PAMOLA’s anonymization and AI-powered policy compliance tools ensure organizations meet evolving regulatory standards, reducing legal exposure while maintaining data utility for cybersecurity analytics.
LLM-Driven Threats & AI Exploitation Risks
Large Language Models (LLMs) introduce new attack surfaces, including data poisoning, adversarial AI manipulation, and unstructured text vulnerabilities. AYITA’s AI security monitoring detects and mitigates AI-driven threats while ensuring explainability and control over enterprise LLM applications.
AI Solutions for Next-Gen Cybersecurity
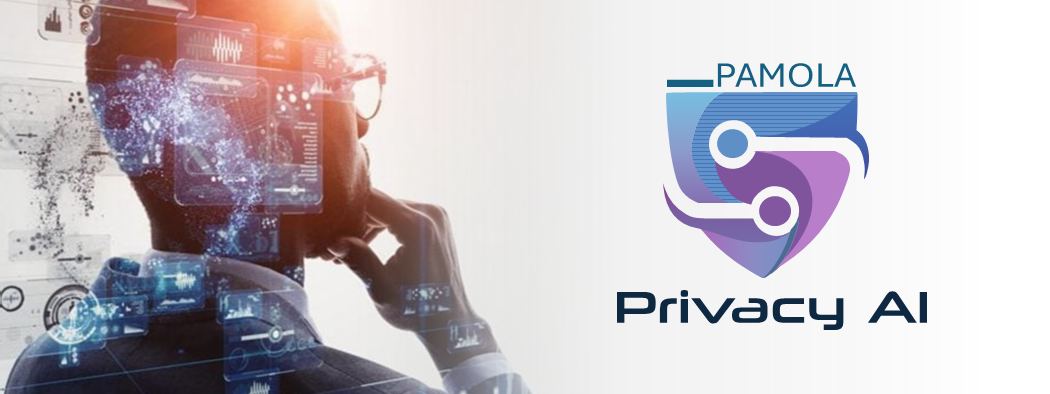
PAMOLA: AI-Powered Privacy & Data Security
In an era of growing data breaches and AI-driven threats, securing sensitive data is more critical than ever. PAMOLA enables organizations to train AI models on encrypted, anonymized, or synthetic data without exposing real information. Using federated learning (FL) and secure multi-party computation (SMPC), PAMOLA allows businesses to collaborate securely without risking data leaks or regulatory non-compliance.
Whether it's protecting enterprise AI workflows, ensuring privacy in cyber threat analysis, or securing cross-organization data collaboration, PAMOLA provides privacy-first AI security solutions.
Learn More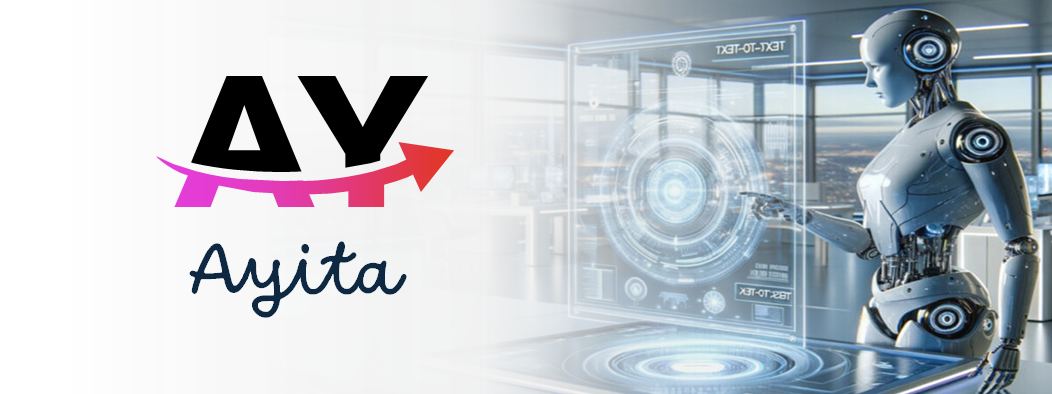
AYITA: AI Security & LLM Risk Monitoring
As Large Language Models (LLMs) reshape cybersecurity landscapes, new risks emerge, including data poisoning, adversarial AI threats, and unstructured text vulnerabilities. AYITA provides **real-time AI security monitoring** to detect and mitigate malicious AI activity, ensuring that enterprise LLM deployments remain resilient and trustworthy.
From analyzing unstructured threat intelligence to automatically detecting AI-generated exploits, AYITA enables security teams to identify risks before they escalate. With its explainable AI engine, AYITA enhances transparency and control over AI decision-making in cybersecurity operations.
Learn MoreCybersecurity AI Use Cases
Secure Data Collaboration Across Organizations
Companies need to share threat intelligence and fraud detection insights while ensuring sensitive data is not exposed. PAMOLA’s federated learning (FL) and secure multi-party computation (SMPC) enable cross-organization AI model training without data leaks, ensuring compliance with GDPR, CCPA, and emerging AI security standards.
- Privacy-First AI Collaboration: Train security models across industries without sharing raw data.
- Regulatory Compliance: Meet data protection laws while improving cyber defense.
- Cross-Sector Intelligence: Securely integrate threat intelligence between financial, government, and tech sectors.
Protecting AI from Data Poisoning and Model Attacks
Attackers increasingly target AI models through data poisoning and adversarial attacks. AYITA’s AI monitoring and explainability tools detect anomalies, prevent model corruption, and ensure that security teams maintain control over AI-driven decision-making.
- AI Threat Detection: Identify malicious data injection attempts.
- Explainable AI Security: Ensure transparency in AI decision-making for security teams.
- Real-Time Model Defense: Automatically flag and mitigate AI model vulnerabilities.
Automated Compliance & Data Anonymization
Data privacy laws are evolving rapidly, making compliance a challenge for security teams. PAMOLA’s automated anonymization and differential privacy ensure that organizations can process, analyze, and share data securely while staying aligned with GDPR, CCPA, and AI transparency laws.
- Regulatory Alignment: Automate anonymization for compliance audits.
- Privacy-Enhancing Analytics: Extract insights from protected datasets without revealing identities.
- Zero-Trust Data Sharing: Enable secure AI-powered risk assessments.
LLM Security & Protection Against AI-Powered Threats
Large Language Models (LLMs) can be exploited for social engineering, automated phishing, and deepfake attacks. AYITA’s AI risk detection and LLM security framework monitors enterprise AI deployments for suspicious patterns, preventing exploitation.
- AI Abuse Monitoring: Identify adversarial prompts and malicious model outputs.
- LLM Exploit Prevention: Protect against AI-driven phishing and misinformation.
- Enterprise AI Governance: Maintain control and explainability over AI-generated content.
AI-Driven Threat Intelligence Processing
Cybersecurity teams must process vast amounts of unstructured threat intelligence data. AYITA’s AI-enhanced NLP models automatically analyze logs, reports, and open-source threat feeds, ensuring security teams get actionable insights in real time.
- Automated Threat Detection: Extract key security indicators from unstructured data.
- Real-Time Intelligence Processing: Prioritize high-risk threats with AI-driven categorization.
- Scalable Security Insights: Improve SOC (Security Operations Center) efficiency with AI-enhanced monitoring.
AI-Agent Based Cyber Defense
Security teams are overwhelmed by **manual threat detection and incident response**. AYITA’s autonomous AI agents operate as **intelligent cybersecurity assistants**, capable of **proactive threat mitigation, AI-driven anomaly detection, and automated attack response**.
- Proactive AI Threat Defense: Deploy autonomous AI agents to counter cyberattacks in real time.
- Automated Incident Response: Reduce response times by allowing AI to execute predefined security actions.
- Adaptive AI Security: Continuously learn from threats and improve defensive capabilities.
Privacy Risks & AI Protection
As organizations adopt AI and big data analytics, new privacy challenges emerge. Attackers exploit AI models to infer sensitive data, re-identify anonymized records, and manipulate training datasets to inject bias or vulnerabilities. PAMOLA and AYITA offer advanced protection against these risks, ensuring secure AI implementation without compromising compliance or data confidentiality.
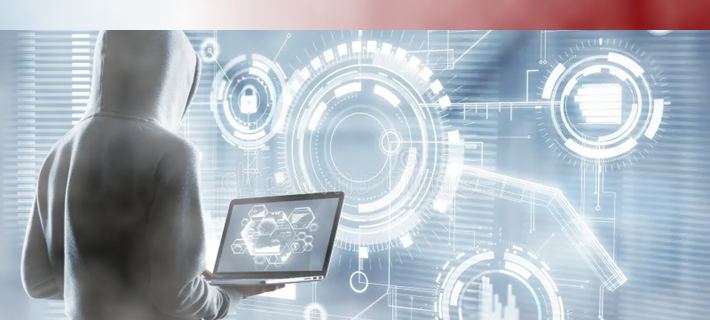
Re-Identification & Single-Out Risks
Even anonymized datasets can be reverse-engineered to reveal an individual’s identity. Attackers analyze **statistical uniqueness** in data to isolate specific records. PAMOLA’s advanced anonymization and synthetic data mitigate these risks by ensuring that sensitive attributes cannot be linked back to real individuals.
- Solution: Differential privacy and k-anonymization reduce re-identification risks.
- Use Case: Protect personal data in medical research and financial transactions.
Inference Risks
AI models can unintentionally **reveal hidden insights** about individuals. Attackers analyze model outputs to infer private attributes, such as health conditions or financial status. PAMOLA’s privacy-preserving AI techniques ensure that sensitive details remain protected during AI training.
- Solution: Privacy-aware AI modeling reduces exposure of hidden patterns.
- Use Case: Secure medical AI predictions without exposing patient conditions.
Linking Risks
By combining **public, leaked, and internal datasets**, adversaries can **connect disparate records** to reconstruct private user profiles. PAMOLA’s federated learning (FL) enables secure AI training without centralized data pooling, minimizing these risks.
- Solution: Controlled data linkage and secure AI collaboration.
- Use Case: Privacy-preserving fraud detection across financial institutions.
Membership Inference Risks
Attackers can determine whether specific individuals were part of an AI model’s training set, potentially revealing confidential participation in medical or financial records. PAMOLA’s differential privacy ensures that training data remains indistinguishable.
- Solution: Noise injection and privacy-aware model training.
- Use Case: Protect AI models in healthcare and fraud detection.
Model Inversion Risks
Attackers use AI models to **reconstruct sensitive training data**, extracting **facial images, medical records, or proprietary datasets**. AYITA’s AI monitoring detects and mitigates these risks through real-time model behavior analysis.
- Solution: Controlled model access and differential privacy.
- Use Case: Prevent unauthorized recovery of sensitive training data.
Data Poisoning & AI Manipulation
Attackers inject **malicious or biased data** into AI training sets, causing models to behave unpredictably. AYITA’s AI threat detection continuously monitors AI model integrity, preventing compromised data from influencing results.
- Solution: AI audit logs, anomaly detection, and adversarial training.
- Use Case: Secure AI-powered cybersecurity tools from adversarial attacks.
Explore Our Privacy & Security Frameworks
Access expert insights on risk management for PETs (Privacy-Enhancing Technologies) and secure transactions in distributed environments.
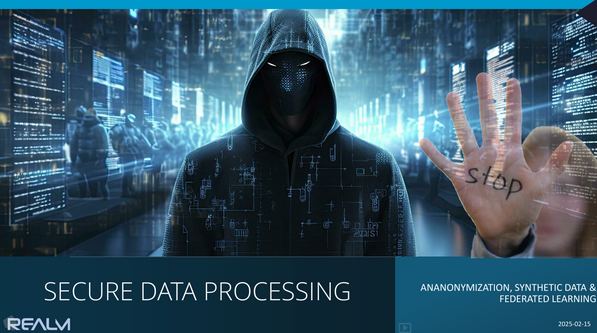
Secure Data Processing (SDP)
A risk management framework for Privacy-Enhancing Technologies (PETs), including risk calculations for anonymization, synthetic data, and federated learning.
Download Brochure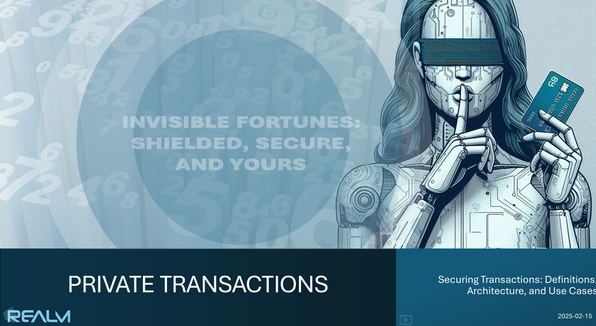
Private Transactions
Learn how Zero-Knowledge Proofs (ZKP) enable privacy-preserving transactions in decentralized environments, ensuring secure and verifiable data exchange.
Download Brochure